Priyadarshini Kumari
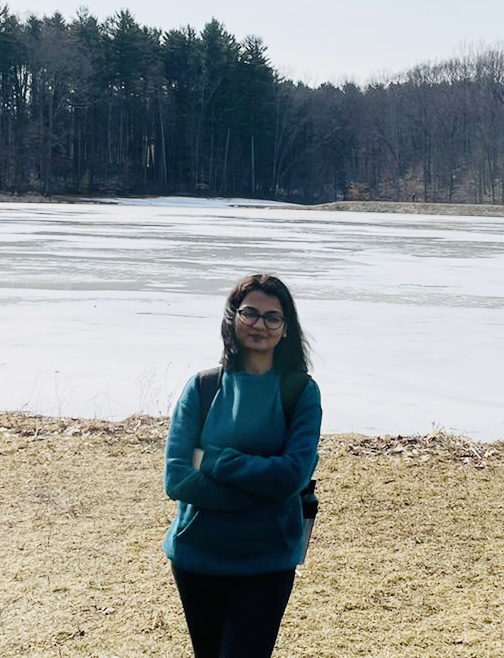
I work at Apple, focusing on the intersection of machine learning and health.
Previously at Sony AI, I contributed to a range of projects, from developing data-efficient machine learning techniques for graph neural networks to creating multimodal perception models integrating text, and olfactory inputs. My work spanned applications in biomedical research, olfaction, and gastronomy.
I received my Ph.D. from IIT Bombay, advised by Prof. Subhasis Chaudhuri and Prof. Siddhartha Chaudhuri. My thesis was on Label-Efficient Distance Metric Learning. Before that, I completed my master’s also from IIT Bombay where I developed multimodal rendering techniques that combined haptic, visual, and auditory feedback to make 3D models of heritage sites accessible to the visually impaired.
Here is my CV
news
Jul 25, 2024 | Our paper “Link prediction for hypothesis generation: an active curriculum learning infused temporal graph-based approach” is accepted at Artificial Intelligence Review 2024. |
---|---|
Jul 12, 2024 | Our paper “CosFairNet:A Parameter-Space based Approach for Bias Free Learning” is accepted at BMVC 2024. |
Oct 31, 2023 | Our paper “FRUNI and FTREE synthetic knowledge graphs for evaluating explainability” is accepted at NeurIPS XAIA workshop 2023. |
Sep 21, 2023 | Two papers “Perceptual metrics for odorants: learning from non-expert similarity feedback using machine learning” and “Comparing molecular representations, e-nose signals, and other featurization, for learning to smell aroma molecules” are accepted at PLOS One |
Jul 15, 2023 | Our paper “Optimizing Learning Across Multimodal Transfer Features for Modeling Olfactory Perception” was accepted to Multimodal SIGKDD 2023 |
selected publications
- Link prediction for hypothesis generation: an active curriculum learning infused temporal graph-based approachIn Artificial Intelligence Review , 2024
We present THiGER-A, a solution aimed at addressing the hypothesis-generation problem. This method utilizes a temporal graph-based node-pair embedding and incorporates an active-curriculum training approach to precisely capture the dynamic evolution of discoveries over time.
-
- Optimizing Learning Across Multimodal Transfer Features for Modeling Olfactory PerceptionIn International Workshop on Multimodal Learning at SIGKDD , 2023
We introduce a novel multilabel and multimodal transfer learning technique for modeling olfactory perception. Our approach aims to tackle the challenges of data scarcity and label skewness in the olfactory domain.
- PerceptNet: Learning perceptual similarity of haptic textures in presence of unorderable tripletsIn IEEE World Haptics Conference (WHC) , 2019
We propose a deep metric learning approach that demonstrates the utility of ambiguous triplets for effectively modeling human perception.